In the era of big data, the ability to draw meaningful insights from vast amounts of information is a valuable skill. Data science and statistical inference are two critical fields that enable professionals to make sense of complex data, driving informed decision-making across industries. A comprehensive data science course can equip individuals with the skills needed to leverage these powerful tools effectively. Together, they form the backbone of data-driven strategies, combining cutting-edge technology with foundational statistical principles. This article explores how data science and statistical inference work together and why mastering both is essential for aspiring professionals.
Top Courses in Data Science Engineering
More Courses With Data Science Certification
Understanding Data Science
In order to derive useful insights from data, data science is an interdisciplinary field that blends computer science, mathematics, and domain experience. Data collection, cleaning, processing, analysis, and visualization are all part of it. At its core, data science aims to transform raw data into a meaningful narrative, enabling businesses to identify trends, optimize operations, and solve problems.
Professionals in data science use a variety of tools and programming languages such as Python, R, and SQL. To create prediction models and get more profound understanding, they utilize machine learning algorithms. A comprehensive data science course can equip learners with the technical skills and knowledge needed to excel in this dynamic field.
The Role of Statistical Inference
A subfield of statistics called statistical inference is concerned with extrapolating characteristics of a population from a sample. It uses probability theory to make predictions and test hypotheses, ensuring that decisions are backed by data rather than assumptions. Statistical inference plays a vital role in understanding patterns, relationships, and uncertainties within datasets.
Key techniques in statistical inference include:
- Hypothesis Testing: Determining whether a claim about a dataset holds true.
- Confidence Intervals: Estimating the range that a population parameter falls within is known as a confidence interval.
- Regression Analysis: Exploring relationships between variables and predicting outcomes.
Statistical inference provides the theoretical foundation for many data science methods, ensuring that analyses are reliable and conclusions are valid.
How Data Science and Statistical Inference Work Together
While data science focuses on leveraging technology to analyze data, statistical inference provides the theoretical underpinnings that validate these analyses. Here’s how they complement each other:
Data Understanding and Preparation: Before analysis, data scientists rely on statistical techniques to explore and clean data. Descriptive statistics help summarize data, while statistical tests identify outliers and anomalies. These steps are crucial for ensuring data quality and preparing it for advanced analysis.
Model Development and Validation: Machine learning models in data science are built on principles of statistical inference. For instance, linear regression, a common predictive modeling technique, originates from statistics. Statistical inference helps in evaluating model performance through metrics such as p-values and confidence intervals.
Uncertainty and Predictions: Data science models often produce predictions, but understanding the uncertainty associated with these predictions requires statistical inference. Techniques like bootstrapping and Bayesian analysis provide insights into the reliability of the results, guiding better decision-making.
Communication of Results: Visualization and reporting are integral to data science. Statistical inference enhances the credibility of visualizations by ensuring that the presented trends and patterns are statistically significant.
Practical Applications of Their Synergy
The collaboration between data science and statistical inference drives innovation and efficiency across various sectors:
- Healthcare: In predictive analytics, data science models forecast disease outbreaks, while statistical inference validates these predictions. Together, they enable personalized medicine and effective healthcare strategies.
- Finance: Financial institutions use data science to detect fraud and assess credit risk. Statistical inference ensures the accuracy of these assessments, reducing false positives and enhancing trust.
- Marketing: Companies analyze consumer behavior using data science techniques like clustering and segmentation. Statistical inference validates the findings, ensuring that marketing strategies are data-driven and effective.
- Technology: In AI and machine learning, data science drives innovation, while statistical inference provides a rigorous framework for evaluating model performance and fairness.

How to Learn Data Science and Statistical Inference
For students and professionals looking to delve into data science, enrolling in a data science course is a great place to start. A comprehensive data science course covers various topics, including data cleaning, machine learning, statistical analysis, and data visualization. Understanding statistical inference is essential, as it underpins many of the techniques used in data science.
By taking a data science course that includes statistical inference training, you’ll gain the skills needed to analyze data accurately and confidently. At easyshiksha.com, there are numerous online resources and courses designed to provide practical knowledge and hands-on experience, allowing students to master both data science and statistical inference.
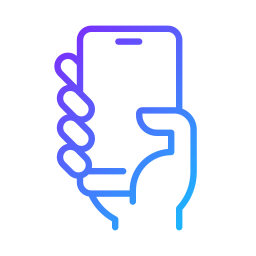
Why Integration Matters
The integration of data science and statistical inference is crucial for making informed decisions in today’s data-driven world. Without statistical inference, data science models risk being biased or unreliable. Conversely, without the technological capabilities of data science, statistical analyses may remain limited in scope and scalability.
Professionals who understand both domains are better equipped to:
- Design experiments and interpret results accurately.
- Build robust predictive models that account for uncertainty.
- Communicate insights effectively to stakeholders.
Check out our latest article: Why Full Stack JavaScript Developers Are in High Demand
Get Courses: microsoft azure machine learning
Conclusion
The relationship between data science and statistical inference is fundamental to extracting meaningful insights from data. Statistical methods enable data scientists to make reliable predictions, test hypotheses, and guide business decisions with confidence. As data science continues to grow, mastering statistical inference will remain a critical skill for anyone looking to excel in this field. By understanding and applying statistical inference, you will be equipped to build robust models and ensure your analyses are both accurate and actionable.
Platforms like EasyShiksha.com make it easier than ever to start this journey, offering accessible and well-structured learning programs. By mastering the synergy between data science and statistical inference, learners can position themselves as valuable assets in the data-driven economy and contribute meaningfully to solving complex global challenges.